How can exhibition-makers make use of existing AI tools?
Stable Diffusion has become a popular AI tool for image generation. Launched in 2022, the text-to-image deep-learning model promises to create detailed images through text commands (prompting). The recent versions, also offer additional options for inpainting (editing the interior of an image) and outpainting (expanding the original image frame).
For exhibition-making, this sort of tool may be useful for visualising exhibition design ideas, serving as inputs for a moodboard, for example. It could also be used to quickly create variations of an existing exhibition design or test out a particular style or composition before developing products in a more appropriate tool.
In practice, this requires a lot of patience and a somewhat developed notion of “effective” prompting. Still, it will certainly require some follow-up edits and customization.
In our attempts to test out those more “accessible” tools for exhibition-making1, we set up a very simple test run by attempting to generate a form of “exhibition space” through, let’s call it, a “beginner-level prompting” (considering that most people engaging with these tools for the first time will not have extensive prompting skills and will use word commands intuitively).
Using an unofficial web tool based on Stable Diffusion, we asked it to generate:
“An exhibition space at the MoMA in 1969 with Picasso artworks in black and white photograph”
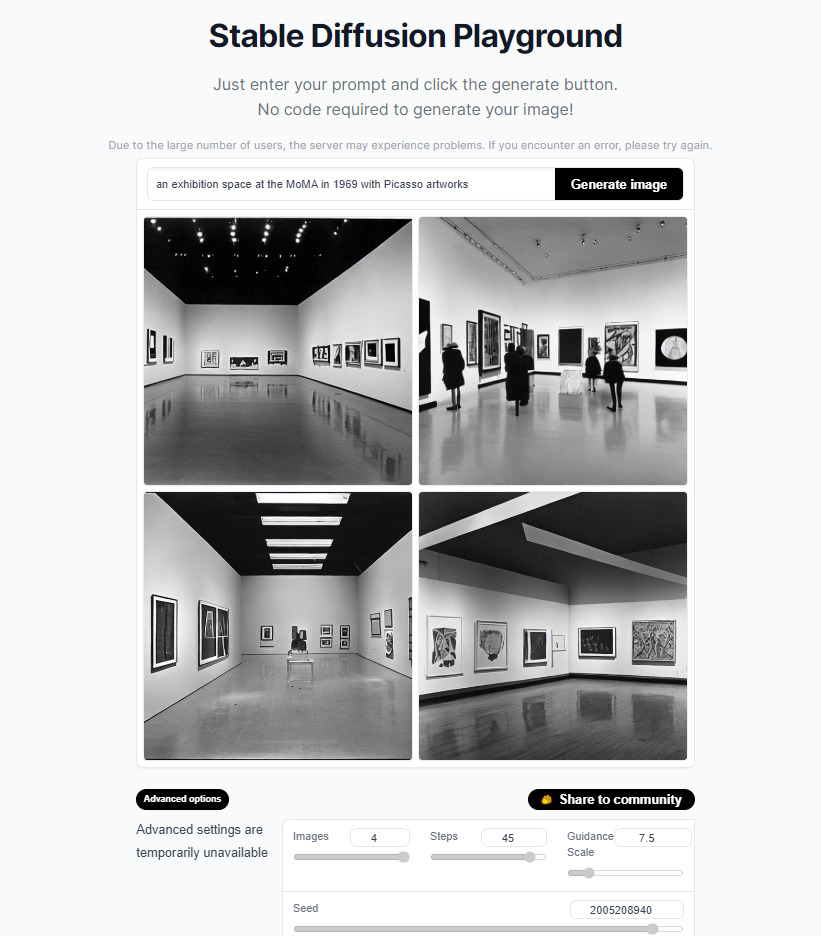
The results were surprisingly effective, considering the simplicity of the prompt, the tool’s available resources, and the expected visual errors regarding ceilings, lighting and human figures. The space created is in fact very similar to what we see in MoMA’s 1960s exhibition views.
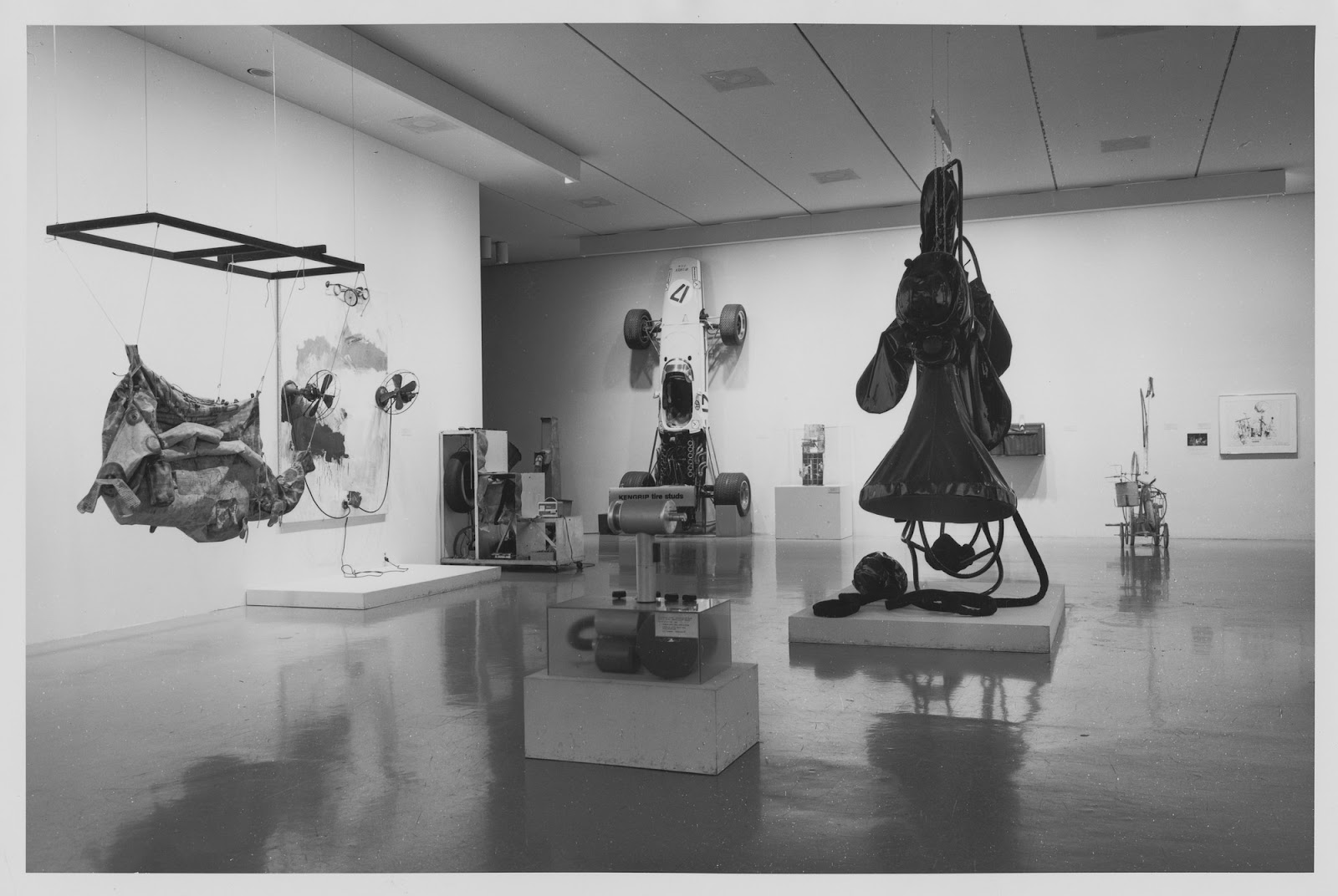
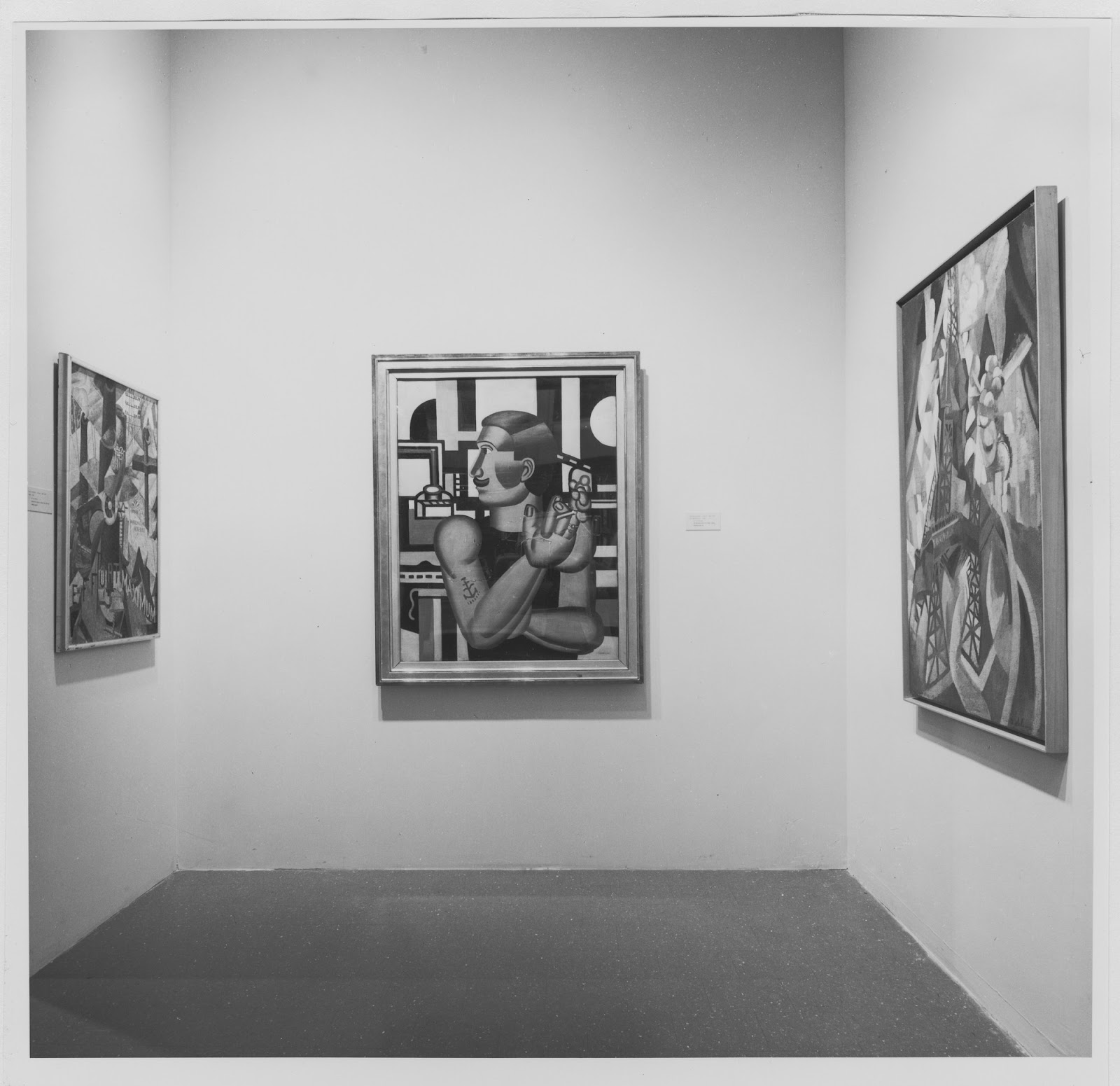
Image credits: The Machine as Seen at the End of the Mechanical Age (1969), exhibition views, MoMA.
However, when asked to create an exhibition space of a lesser-known museum with a more generalistic type of exhibited object, the outcomes were disappointing, highlighting the need for more complex prompting.
“An exhibition space at the MUHNAC in Lisbon in 2020 with science objects”
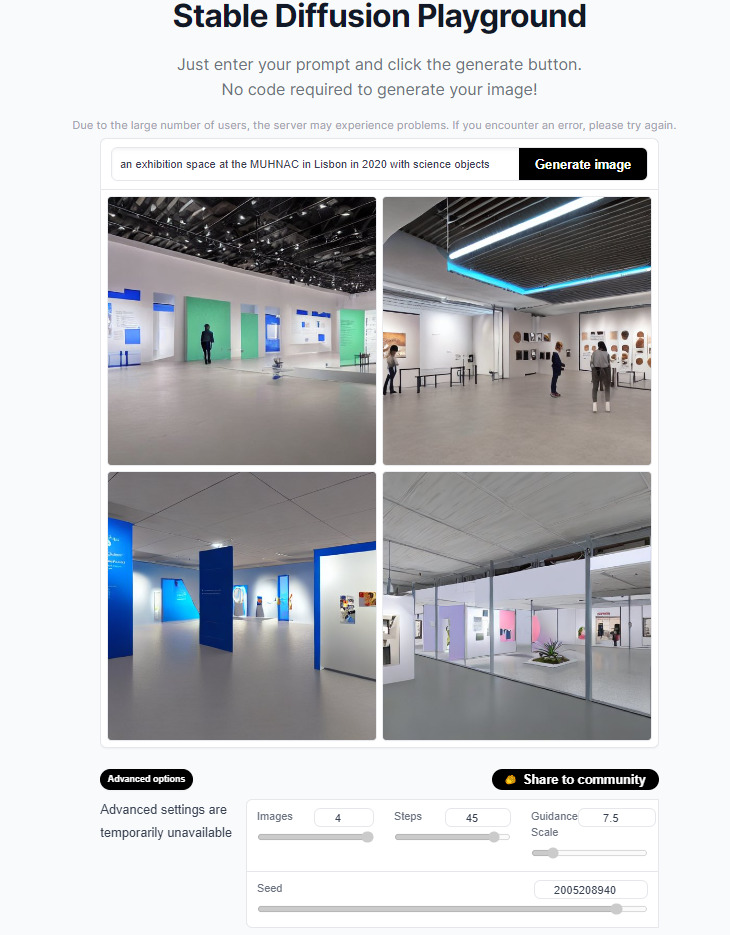
Even if this simple prompt had a bit more explanation to it (let’s say, by specifying a “natural history museum” type), the case already makes evident the significance of the data these models are trained on (and already extensively addressed in literature and media).
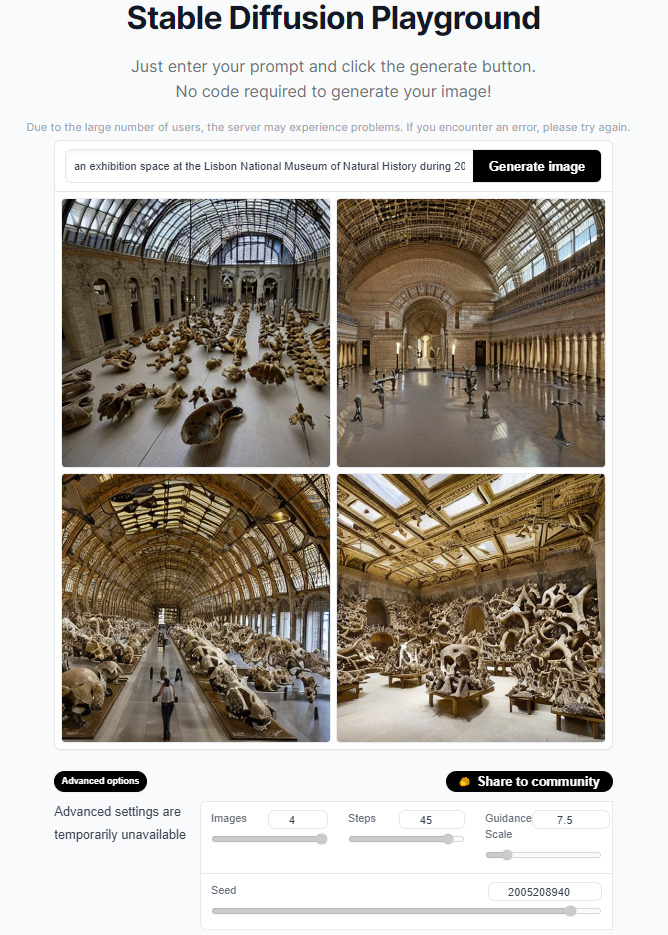
What is then interesting for museums is perceiving what sort of visual imagery these tools produce when it comes to representing what a museum and an exhibition space “look like” and what this can tell us about the tool’s limitations and the unrealistic idea of their actual capabilities. Moreover, it suggests that one does not need to be tech-savvy or actively engaged with the current debates to be able to assess the limitations and issues these tools bring into discussion. Those discussions can become much more fruitful if confronted with already existing debates in museum practice, such as those on archival data biases, museum and exhibition definitions and roles, and the importance of spatial elements in the development of particular environments for cultural institutions.
- Stable Diffusion, however, is not the most accessible one, as it demands a bit more horsepower and sometimes a working knowledge of gits and an online community culture savviness to successfully run it at a local level, that is, instead of using the online version through Stability AI’s DreamStudio, which requires paid credits, one can use it for free through a community developed web-like interface such as Automatic1111’s webUI. There are, however, one-click install options.